Predicting the Unpredictable: Zapata AI Accurately Predicts the Future in Real-time on the Sensor Intelligence Platform
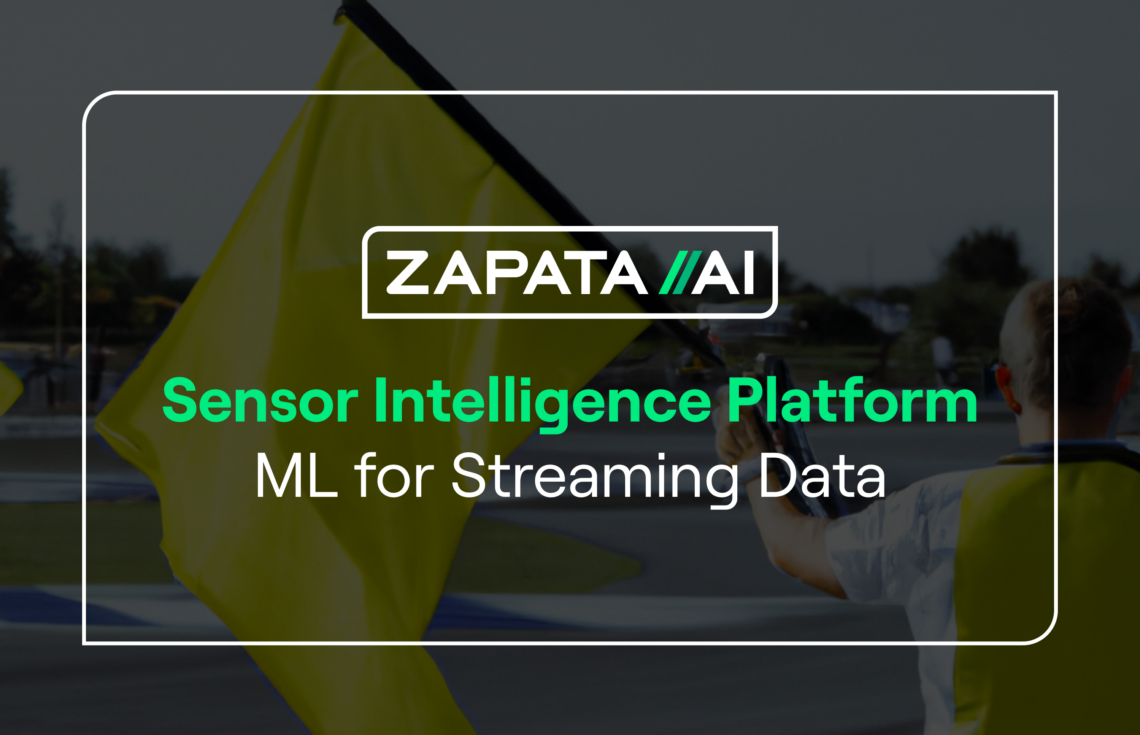
Predicting the Unpredictable: Zapata AI Accurately Predicts the Future in Real-time on the Sensor Intelligence Platform
Sudden, unexpected disruptions are costly in any industry — whether it’s a telecom service outage, an equipment failure in manufacturing, or a market dip in financial services. Predicting these disruptions ahead of time and preparing a mitigation plan allows enterprises to avoid inevitable costs and delays to their operations and provides an opportunity to take action before the competition.
Predicting the future. No problem, right? Of course, that’s easier said than done. With thousands or even millions of nodes in a large operational network emitting torrents of sensor data, enterprises face the challenge of making sense of all this data, cleaning and processing it, and identifying patterns and clues along the way which could portend a major disruption is coming.
This is as true in INDYCAR® racing as it is in any other industry. By leveraging generative AI, machine learning, and advanced optimization in Zapata AI’s Sensor Intelligence Platform (SIP), we are helping our customer Andretti Global ingest live streaming data from sensors on cars and around the racetrack, coupled with models trained on performance data from past races, to predict disruptive yellow flag events in real-time during a race — in an effort to give them a critical race strategy advantage.
We’re in the thick of the INDYCAR® racing season, and the Grand Prix of Long Beach is upon us. See for yourself – a demonstration of Zapata AI’s yellow flag prediction capability, drawn from Andretti’s performance data in a 2024 INDYCAR® race, can be found below:
But, you may ask, why do yellow flags matter?
In auto racing, yellow flags can be some of the most disruptive events that can happen during a race, forcing unexpected and immediate decision making from race teams — decisions which often have a significant impact on winning or losing.
A yellow flag is flown after an accident, collision, or as other hazardous conditions present themselves on the track, forcing all drivers to significantly reduce their speed and form a tight pack until the hazardous condition is resolved, the damaged car or debris is cleared from the track and it’s safe to return to normal racing. Drivers who remain on the track must drive very slowly, remaining in the same racing order as the moment when the yellow flag was flown (e.g., no passing or competitive moves are allowed).
A key strategic challenge is that during yellow flags in INDYCAR®, the pit lane closes until all the drivers slow down and can bunch up, and only then does the pit lane re-open. Drivers caught in the pits when a yellow flag comes out risk facing drive-through penalties or having to move to the back of the pack.
Often, there are multiple yellow flags during the course of a typical race, and these are usually completely unpredictable. These disruptions have a severe impact on a team’s race strategy — and can throw meticulously developed race plans into chaos, forcing teams to react in the heat of the moment. The question is — what to do?
In auto racing, yellow flags can be some of the most disruptive events that can happen during a race, forcing unexpected and immediate decision making from race teams — decisions which often have a significant impact on winning or losing.
After a yellow flag, a driver who waits for the pack to form up and only then makes a pit stop to change tires and top up his tank is at a huge advantage — they won’t fall as far behind their competitors if everyone is driving slowly and in a pack. Conversely, a driver that pits a few laps before a yellow flag may have unknowingly wasted a pit stop and will fall further behind other drivers than they needed to. Every car must pit several times during a race, and strategically timing these stops can decide who will win or lose a race.
If predicting when an accident or an anomalous, disruptive event will occur during a race sounds complicated and well, unpredictable — that’s because it is. Historically, racing teams have relied upon their intuition, lifelong experience, and “tribal knowledge” to predict when a yellow flag will occur and what to do when the yellow flag flies. Today, race teams have access to terabytes of live streaming sensor and telemetry data from around the racetrack and the cars themselves over the course of a race season, including massive amounts of performance “ground truth” data from previous races over many seasons.
Race teams can crunch that data looking for patterns, signals, or triggers — any actionable insights that would indicate that an anomalous event may be coming up. The challenge is, how quickly during the heat of a race the teams can make sense of all this data, clean, organize, and process it, draw insights from it, and make the best decision. With INDYCAR® lap times ranging anywhere from 20 seconds to 120 seconds depending on the course, these critical decisions — like whether the driver should pit or not — need to be made in a matter of seconds.
Working closely with the Andretti team, Zapata AI deployed the Sensor Intelligence Platform (SIP) to ingest vast amounts of streaming sensor and historical data, turning it into a live prediction of the probability of a yellow flag within the next five laps.
As live data streams into the platform, each new signal triggers a series of data processing activities. This data cleaning and processing is critical to the success of the predictive models on SIP. First, a set of relevant factors, such as traffic density, are calculated based on the raw processed data. These factors are higher level, more informed abstractions which make the raw data usable. They also help with explainability, letting race strategists know why the model predicted what it did and helping build trust in the model.
In SIP, five machine learning models run concurrently, and are continuously being fed by newly processed data and the factors above, with each model focused on the probability of a yellow flag event in each of the next five laps. A new inference model is run based on the latest actual data for every new lap, about every minute. Each of these models are trained on historical race data, looking for correlations in the data with past yellow flag events.
Once all of these predictions are calculated, the results are published to a queue for any subscribing services to pick up and display — in this case, a live dashboard viewable by Andretti race strategists, with the probability of a yellow flag for each future lap. On an ongoing basis, yellow flag predictions from historical races are evaluated to improve the models. All of the data processing and computation is run trackside on the edge of the network, in Zapata AI’s Race Analytics Command Center (RACC).
As you can see in the recorded video, Zapata AI’s Sensor Intelligence Platform accurately predicted multiple yellow flag events well before they occurred during a 2024 INDYCAR® race, giving race strategists critical time to decide what to do before the actual yellow flag was flown.
In addition to predicting yellow flags, Zapata AI is using the same live streaming data processing capabilities, coupled with machine learning, generative AI, and advanced optimization techniques, to help Andretti with car setups, predicting tire degradation, predicting lap times for other cars in the race, as well as repeatedly running optimization algorithms to inform strategic decisions during the race.
The value of predicting anomalies and disruptions in real-time in challenging, dynamic environments is not limited to auto racing. This set of capabilities around time series predictive modeling is central to mission-critical, industrial use cases and applications in telecom, finance, transportation & logistics, manufacturing, defense, and more.
The value of predicting anomalies and disruptions in real-time in challenging, dynamic environments is not limited to auto racing.
The same predictive modeling technology Zapata AI uses to predict yellow flags could be applied to detect anomalies, help predict and prepare for unusual events that fall outside normal patterns of operational behavior in industrial settings.
This includes training and running our predictive models on network nodes, sensors and activity logs to predict and mitigate potential service outages, cyberattacks or infrastructure failures for service providers like telcos, utilities, airlines and logistics companies. It could also be applied to analyze unusual financial transaction or communications data to detect potential credit card and banking fraud. Zapata AI’s SIP could also assist human operators in identifying and predicting “black swan” events or anticipating security threats in contested environments with limited or challenging connectivity.
Anomalies are critical for companies to anticipate, predict, and proactively mitigate in every industry — these are just a few examples where Zapata AI could have an impact.
As important as model accuracy, processing speed, and immediacy is, equally important is for the model output to be deployed in an enterprise application that enables operators and decision-makers to quickly and easily interpret and act on these predictions. These insights give leaders a much-needed window to prepare and plan to adapt their operations for the coming disruption — and even prevent it if possible.
Zapata AI’s Sensor Intelligence Platform (SIP) handles the hard part of ingesting and processing immense volumes of live and historical data required to make these predictions — and provides an ideal environment for testing and deploying machine learning and generative AI models that run on this data. SIP also works on the edge, in the most challenging environments, allowing it to run in industrial scenarios with limited compute resources.
Critically, the output from SIP integrates seamlessly, and can be presented in any enterprise application or made available via API as a service call, which allow operators and decision makers the ability to predict the unpredictable — and act on this output.